How IBM can increase employee retention rates ?
- Nghi Truong
- Jan 31, 2020
- 4 min read
The project was a part of our Data Scientist course at Duke Fuqua Master of Quantitative Management (MQM): Business Analytics program, in a collaboration with my classmates Weiqiao Bi, Tianpeng Yu, Tad Kapustiak and Guan-Lun Liao
Introduction
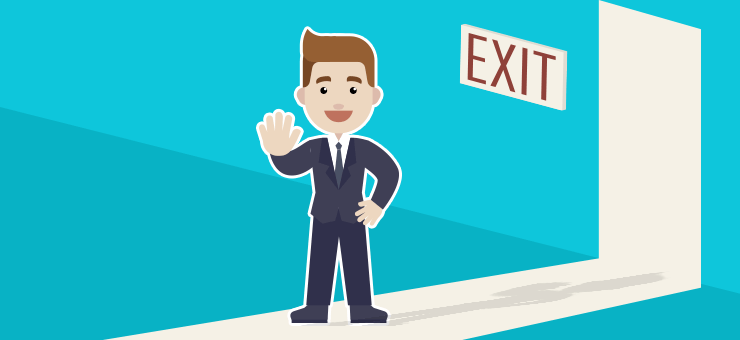
The problem we are attempting to get a better understanding of is attrition at IBM based on a variety of professional and personal factors. If an individual is likely to leave and IBM knows they can either 1) take steps to help ensure that the individual will stay or 2) begin the process of looking for a replacement. Understanding what types of people are likely to churn could also be a useful application of a model like this. However, several of the variables in our model can only be obtained after an individual starts working at the company. Because of this, we decided to focus on predicting the churn rate of individuals already working at IBM. However, if desired, the model could be adapted to focus more on the hiring process to limit the churn rate. The main step to better understand attrition at IBM is regression analysis, mainly regression analysis in the form of Lasso, Post Lasso, and Logistic Regression. All of these models are supervised data mining with the express goal of trying to predict the chances that an individual will churn.
Dataset
We used Kaggle IBM HR Analytics Attrition as main dataset which included 1470 observations with 35 columns.
Exploratory Analysis

Based on Graph 1, we can see that the hourly rate among attrition groups are similar, which means that hourly rate does not have much impact on attrition decision. And Graph 2 shows turnover rates are different among different job roles

From Graph 3. shown below, attribution has a negative correlation with overtime. If the employee work overtime, he/she will have a higher possibility of churn. For the work-life balance as Graph 4., if the employee has a very low level of work-life balance, he/she will have a higher chance of churn.

We aslo conducedt Unsupervised Learning (clustering) to better understand our data.
In Graph 5., Employees in the company could be classified into 4 clusters: 1). Acua is the recently-hired, 2). Blue is junior employees who haven’t been promoted , 3). Red is the senior employees who just got promoted, 4). Green stands for senior employees who haven’t been promoted for a while. In Graph 6., we can see the MonthlyIncome is not positively correlated to TotalWorkingYears perfectly, the cluster in Green is people with multi-years of work experience but having a comparably low monthly income, a group we think might have higher chance to churn.
Modelling
Features Selections
In order to make reasonable variable selections, we firstly used Lasso and Post Lasso to select variables. For both Lasso and Post Lasso, we chose three lambdas to select variables. The first is lambda with minimum deviance, the second is lambda with one standard error away from minimum deviance lambda and the third is lambda from Lasso theory. We also tried Post Lasso by constructing three logistic regression models with lambdas we used in Lasso. The minimum lambda selected 36 variables, the lambda which is one standard error from the minimum selected 27 variables, the lambda provided by Lasso theory selected 16 variables. We also included the null model, normal logistic regression and normal logistic regression with interactions in comparison. We used out-of-sample k-fold ACC and out-of-sample K-fold R as two performance metrics to compare the nine models
Evaluation:
From the intuitive model validated by k-fold method, we generated an average R squared of 0.15 and an average ACC of 83%. For Lasso, Post Lasso, Logistic, Logistic with interactions and Null, we can see that Post Lasso with one standard error has highest average ACC as well as average R-squared compared to other models. However, as Post Lasso with one standard error chose 27 variables in mode, the K-fold experience is not very stable. ACC ranged from 82% to 91% with an average of 87% and 90% the ACC greater than 84% of the null model while of R-squared ranged from 0.16 to 0.39. We decided two use Post-Lasso with one standard error as our final model as the accuracy is the most important metric of binary classification

Final Models
The probability of attrition based on Post-Lasso model is given by looking at significant level of these factors in logistic regression
Attrition = (Basic Information) 6.1 -0.03*Age +0*DailyRate +0.42*Male -0.79*Married -1.05*Divorced (Education Background and Job Role) -0.81*DepartmentResearchDevelopment +0.36*EducationFieldMarketing +0.93*EducationFieldTechnical.Degree +1.01*JobRoleLaboratory.Technician -0.82*JobRoleResearch.Director +0.65*JobRoleSales.Representative (Professional Experiences) -0.05*TotalWorkingYears +0.19*NumCompaniesWorked +1.98*OverTime -0.11*YearsWithCurrManager -0.19*TrainingTimesLastYear (Satisfaction Level) -0.54*JobInvolvement -0.41*JobSatisfaction -0.36*WorkLifeBalance -0.24*RelationshipSatisfaction -0.43*EnvironmentSatisfaction (Job Level and Promotion) -0.2*JobLevel +0.16*YearsSinceLastPromotion -0.13*YearsInCurrentRole (Other Factors) -0.24*StockOptionLevel +0.96*BusinessTravelFrequency +0.05*DistanceFromHome (Clustering) -1.06*cluster2 -0.78*cluster3 -1.71*cluster4
Based on this model, we can see some group are more sensitive than other group in attrition. Firstly, overtime is the factor with the highest significant positive coefficient (1.9811), proving that employees with overtime is more easily to leave the companies. Secondly, EducationFieldTechnical.Degree and JobRoleLaboratory.Technician have second largest positive coefficients, indicating that people with technical background have more attrition propensity than people in marketing or sales. Moreover, people with high distance from home and more frequent travel are more likely to resign from companies. Also, compared to new employee (cluster1), junior employee group without promotion (cluster 2) are more likely to leave the companies which will cause a huge loss for companies as these groups are well-trained, understand companies procedure and can perform their tasks skillfully compared to younger group. On the other hand, married or divorced people are more likely to stay with company compared to single person. Also, by providing more training to employees, improving working environment as well as job design, companies can reduce attrition propensity. Surprisingly, daily rate or monthly rate have no significant impact on attrition, proving that increasing salary does not help companies to keep people.
Comments